The Role of Synthetic Data in Enhancing Analytical Models and Protecting Sensitive Information
In a world where data is king, the ability to create and manipulate vast amounts of information is becoming a game-changer for industries ranging from healthcare to finance. But what if the data you need doesn’t have to be collected from the real world? We are, of course, referring to the concept of synthetic data - a powerful and flexible tool that’s rapidly reshaping how organisations train AI models, test systems and protect sensitive information. But what exactly is synthetic data, and how does it work? More importantly, why is it becoming so crucial for businesses striving to stay competitive in the era of big data?
What is synthetic data?
Synthetic data is artificially created information that mimics real world data but is generated algorithmically rather than collected from actual events. Using algorithms, it is generated to serve as a substitute for actual data, particularly in situations where large and high quality datasets are difficult, expensive or time consuming to obtain. This makes synthetic data especially valuable for testing machine learning models or validating mathematical systems.
As organisations increasingly seek to leverage big data, the demand for synthetic data is growing, with predictions from the likes of Gartner stating that up to 60% of the data used for AI and analytics in the near future will be artificially generated. One of the primary benefits of synthetic data is its flexibility - it can be produced in virtually unlimited quantities and tailored to fit specific needs. For example, training machine learning models often requires large, well-labelled datasets, and synthetic data can provide this at a fraction of the cost of gathering and labelling real data. It also helps address privacy concerns, as synthetic data can mimic real world datasets without containing personal or sensitive information. Additionally, it can be used to reduce bias in data and ensure that diverse datasets are available. Various techniques, such as generative models and agent-based simulations, are employed to create synthetic data, each capable of producing realistic, high quality data for different applications.
How does it work?
Synthetic data is generated through various methods and techniques, each tailored to specific use cases. Here are some of the most common methods:
One of the most popular approaches is using Generative Adversarial Networks (GANs). This is one of the most popular approaches and involves producing AI-generated content by training two neural networks against each other. Another common method involves randomly drawing numbers from a distribution, which can mimic real world data patterns without necessarily capturing the underlying insights. A more advanced approach is agent-based modelling, where individual agents, like people or computer programs, interact within a system to simulate complex real world dynamics. This method is especially useful for studying behaviours in fields such as telecommunications or crowd simulations.
These models analyse statistical patterns in real data, learn from them and then generate new data with similar characteristics. This allows for the creation of realistic, synthetic data that retains the structure of the original data while being completely artificial. Applications of synthetic data span numerous industries, including finance, healthcare and autonomous driving. For example, Google’s Waymo uses synthetic data to train self-driving cars, while JPMorgan employs it for fraud detection.
Synthetic data can also take many forms, from text and tabular data to more complex, unstructured data like images and video. Tools like DALL-E can generate highly realistic images from text descriptions, showing how versatile synthetic data can be. Additionally, synthetic data allows for enhanced creativity and flexibility. For instance, it can generate different views of an object based on a single image, offering perspectives not possible with real data alone.
The impact of synthetic data on analytical models
Synthetic data is transforming the field of artificial intelligence and machine learning by providing an efficient, customisable and cost effective solution for model training. One of its primary uses is in training neural networks, where vast amounts of carefully labelled data are needed to develop high performing models.
When it comes to model training, synthetic data often outshines real world data in a few key ways.
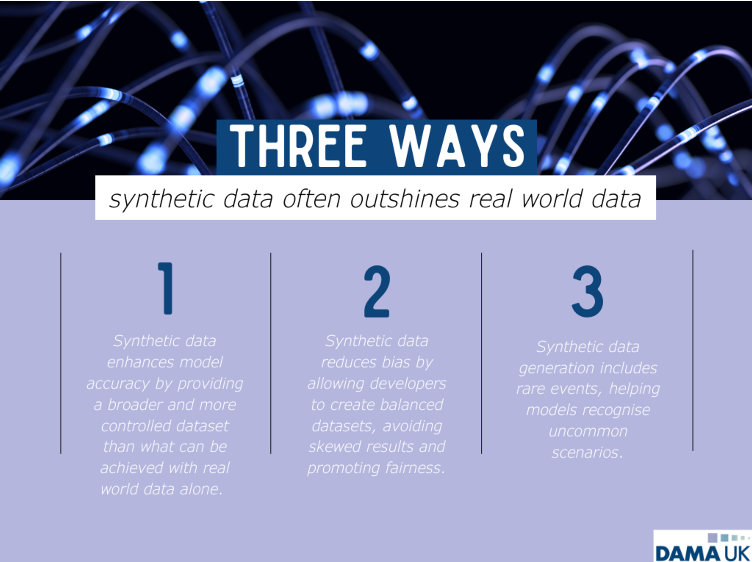
This flexibility makes it invaluable for developing more diverse, fair and explainable AI models. Its adaptability also allows organisations to tailor datasets to specific conditions, which is particularly useful in software testing and quality assurance environments. In terms of cost, synthetic data is a much cheaper alternative to real world data, saving industries such as automotive and healthcare significant resources. Additionally, synthetic data comes pre-labelled, eliminating the time consuming and error prone process of manual labelling, which accelerates model development. Full control over the data’s properties, such as noise levels and event frequency, gives machine learning practitioners a powerful tool to fine tune models as needed.
However, synthetic data does have limitations. It cannot fully replicate the complexity of real data, and authentic data is still essential for creating accurate synthetic counterparts. Despite these challenges, synthetic data is considered the ideal "fuel" for AI and machine learning projects. Furthermore, because synthetic data is artificially generated, it offers complete privacy compliance, ensuring sensitive data remains protected while enabling analysis.
Synthetic data and privacy
Synthetic data offers a powerful solution for protecting user privacy, particularly in industries dealing with sensitive information like healthcare and financial services. By using artificial data that mimics real world datasets without representing actual individuals, organisations can comply with stringent privacy laws such as the Health Insurance Portability and Accountability Act (HIPAA), the General Data Protection Regulation (GDPR) and the California Consumer Privacy Act (CCPA). This makes synthetic data ideal for scenarios where personal data must be safeguarded.
One of the significant advantages of synthetic data over traditional anonymisation or data masking techniques is its ability to eliminate the risk of reidentifying individuals. Unlike pseudonymised data, which still retains traces of personal information and requires legal protection, synthetic data is generated independently of real world users, making it far more privacy compliant. This approach is especially beneficial in healthcare, where researchers can extract useful insights from patient data without violating privacy rules or risking exposure of personal medical records.
The financial sector, too, relies heavily on customer data for internal processes like software testing and fraud detection. Synthetic data allows companies to maintain the statistical properties of real data without breaching privacy regulations, thus enabling smoother data analysis while bypassing long approval processes associated with accessing sensitive information. Additionally, synthetic data fosters greater cross-industry collaboration. By creating datasets that preserve the essence of the original data without revealing personal details, companies can safely share insights across sectors, creating for themselves new opportunities for innovation and economic growth.
Are there any negatives of using synthetic data?
Like all things, there are some downsides to using synthetic data, despite its many advantages. Let’s explore them briefly below:
One of the primary challenges is that synthetic data often fails to fully replicate the complexity and nuances found in real world data. While generative models can produce highly realistic data, they may overlook rare or unexpected patterns that could be critical for certain analyses. Additionally, synthetic data is not a complete substitute for authentic data; real world data is still needed to train the generative models and validate the accuracy of the synthetic outputs. Another drawback is that errors in the generation process, such as poorly trained models or inappropriate parameter settings, can lead to synthetic data that misrepresents the actual conditions it’s supposed to mimic. This can introduce bias or inaccuracies into analytical models. Lastly, synthetic data raises concerns around potential misuse, especially in areas like deepfakes, where artificially generated content can spread misinformation or be used for malicious purposes. So, while synthetic data is a powerful tool, it must be used with caution and in conjunction with real world data to ensure its effectiveness and ethical use.
The takeaway
Synthetic data is opening up exciting possibilities for innovation across industries, offering a cost effective, flexible and privacy conscious solution to the challenges of working with real world data. While it's important to acknowledge its limitations, the advantages far outweigh the drawbacks. With its ability to enhance AI models, reduce bias and generate diverse datasets tailored to specific needs, synthetic data is proving to be a powerful asset in the development of next generation technologies. As it continues to evolve, synthetic data will undoubtedly play a pivotal role in accelerating advancements in AI, analytics and beyond, driving progress and opening new doors for creativity and efficiency.
Sources:
https://www.techtarget.com/searchcio/definition/synthetic-data
https://mostly.ai/what-is-synthetic-data
https://research.ibm.com/blog/what-is-synthetic-data
https://mitsloan.mit.edu/ideas-made-to-matter/what-synthetic-data-and-how-can-it-help-you-competitively